Mapping of large-scale forest coverage by remote sensing can enhance research and applications in the fields of forest resource management, ecosystem protection and restoration, sustainable development, and forest carbon sinks. Addressing challenges such as low consistency among existing remote sensing products for forest coverage and poor spatiotemporal comparability at the pixel scale, led by Prof. Pang Yong, the research team from the Research Institute of Forest Resource Information and Techniques, Chinese Academy of Forestry, has developed a remote sensing image synthesis algorithm that takes phenological characteristics into account. They proposed a technical approach for product aggregation and improvement, enhanced forest coverage mapping accuracy through automatic construction of sample libraries, machine learning recognition, and time series consistency verification, and realized high-precision remote sensing mapping in large areas.
The team has developed a series of 30m resolution remote sensing data products for Chinese forest coverage in 2000, 2010, and 2020.
Utilizing multi-temporal remote sensing images, they further investigated fine-grained classification methods of tree species. Taking larch as an example, the team produced a distribution map for Chinese larch. By integrating airborne lidar data and high-resolution satellite remote sensing data, the team has also developed a remote sensing stratification estimation method for forest growing stock, and a 20m resolution remote sensing data product for Chinese larch biomass in 2020. The results have been published in journals such as GIScience & Remote Sensing and Geo-spatial Information Science, while the data products have been shared at the National Forestry and Grassland Scientific Data Center.
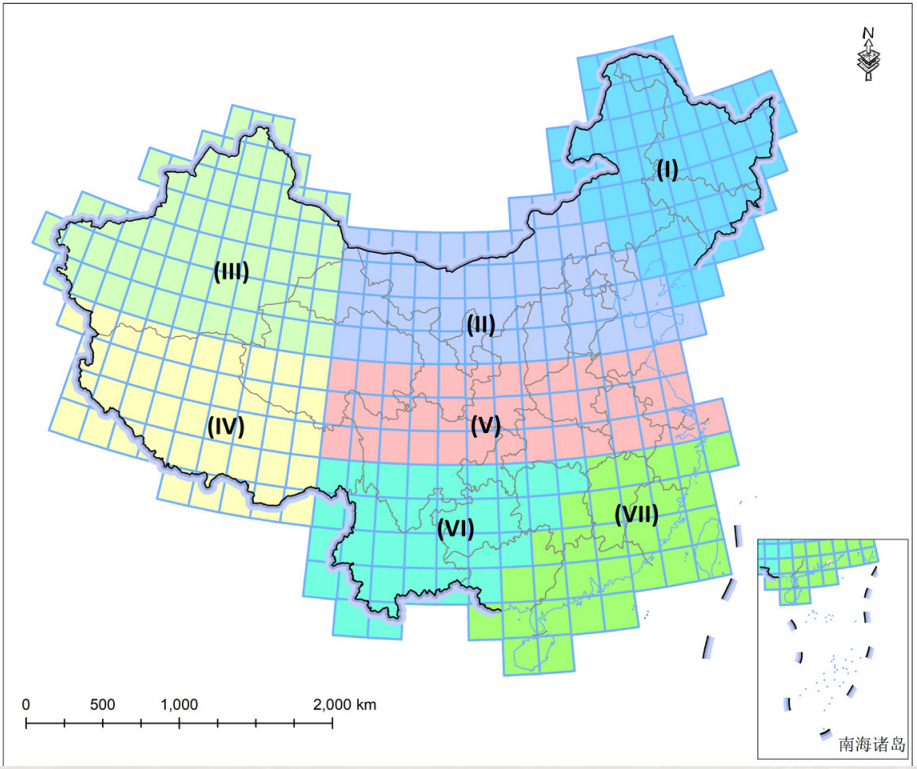